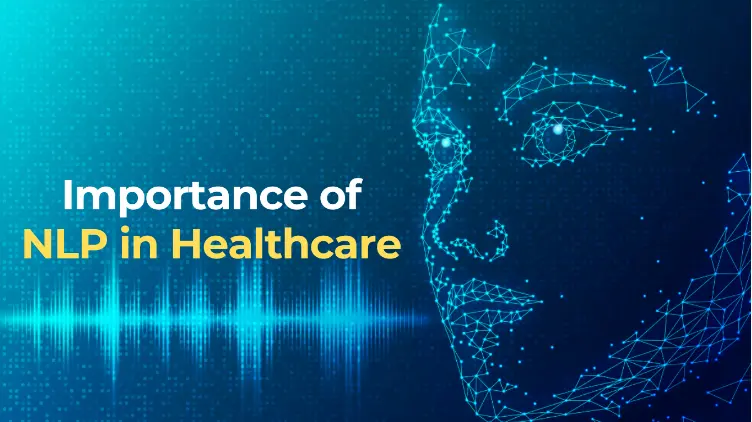
What is the importance of NLP in healthcare?
Table of Contents
ToggleWhat is the importance of NLP in healthcare? Complete Guide
Identifying ailments, creating strategies for therapy & enhancing the experience of the patient.
These represent some potential uses of natural language processing (NLP) in the medical field. To give patients complete treatment and to make sense of the vast amounts of unstructured data included in electronic health records (EHR), an increasing number of healthcare practitioners and providers are implementing natural language processing through leading NLP development services.
We’ll examine natural language processing (NLP) in the healthcare sector in greater detail in this blog article, including its definition and more.
What is NLP & How Does It Work?
Computers can understand and interpret human speech with the help of artificial intelligence called natural language processing.
This is how NLP systems operate: they first “clean” the dataset to pre-process it. This entails arranging the material in a more logical manner, such as tokenization, which divides the text into smaller semantic components, or “tokens.” Pre-processing merely facilitates the NLP system’s interpretation of the dataset.
The system then interprets the text by applying algorithms to it. Rule-based systems, which read text according to predetermined grammatical rules, deep learning services and machine learning models , which employ statistical techniques and “learn” over time by being given training data, are the two main algorithms used in natural language processing (NLP).
NLP is more widespread than you may think, even though it represents a significant scientific breakthrough at the nexus of linguistics and computer science. NLP is used whenever you communicate with a chatbot or an at-home virtual assistant like Siri or Alexa, or when you describe a customer service issue to one of these systems.
5 NLP Techniques You Should Know
A few essential NLP techniques must be defined before we can discuss how NLP can be used in the healthcare industry:
Optical Character Recognition (OCR)
The process via which a computer “reads” handwritten or printed text and transforms it into a digital format is known as optical character recognition, or OCR. For instance, a physical document can be scanned and converted into a PDF. OCR can also be used to scan unstructured data collections, including text files or photos, extract tables and text, and then present the data in an easy-to-read style. This data can be placed into an NLP pipeline for additional analysis after it has been prepared. Clinical notes, medical history data, patient intake forms, discharge summaries, medical tests, and more are frequently digitized in the healthcare sector using OCR.
Named Entity Recognition (NER)
NER is an information extraction method that divides named entities, or actual subjects like a person, place, business, or product, into pre-established groups. Other names for NER include entity identification, entity chunking, and entity extraction. Further down the page, we’ll look at a few NER applications tailored to the healthcare industry.
Sentiment Analysis
To determine a text’s underlying sentiment, sentiment analysis combines natural language processing (NLP), text analysis, computational linguistics, and biometrics. As a result, sentiment analysis is also frequently called opinion mining or sentiment detection.
Text Classification
This NLP technique, sometimes referred to as text categorization, analyzes text data and employs predetermined categories to provide tags or labels to various semantic units or phrases. For instance, depending on certain keywords or phrases found in their medical records, a healthcare professional may utilize text categorization to identify patients who are at risk.
Topic Modeling
To find semantic structures, or “topics,” collections of texts are grouped using common words or phrases. This process is known as topic modeling, and it combines statistical modeling and natural language processing. Latent Dirichlet allocation, the most popular type of topic modeling, groups words and phrases based on their semantic links using algorithms.
OCR and NER are the most widely used NLP techniques in the healthcare sector out of the five that are discussed here.
How Can NLP Support the Healthcare Industry?
Even if there are countless ways that healthcare IT solutions have used NLP, let’s examine three main applications:
1. Improving Clinical Documentation
Instead of wasting time going over complicated EHRs by hand, NLP leverages structured data entry and speech-to-text dictation to extract important information from EHR at the point of service. This guarantees accurate and current clinical documentation while also freeing up doctors to concentrate on giving patients the critical care they require.
2. Accelerating Clinical Trial Matching
Healthcare professionals can automatically evaluate vast amounts of unstructured clinical and patient data and find qualified participants for clinical trials by using natural language processing (NLP). This promotes innovation in the medical sector in addition to giving patients access to experimental care that may significantly improve their health and lives.
3. Supporting Clinical Decisions
At the point of care, NLP helps doctors make better decisions by providing them with quick, simple, and effective access to health-related information when they need it.
4. Medical Research
NLP processes scientific literature and identifies relevant studies, saving researchers significant time. It also helps in meta-analyses and systematic reviews by quickly summarizing findings from large datasets.
5. Patient Interaction
Chatbots and virtual assistants powered by NLP provide instant support for patient inquiries. They assist in appointment scheduling, answering FAQs, and providing reminders for medications or follow-ups.
6. Drug Development
NLP accelerates drug discovery by analyzing clinical trial data, identifying patterns, and predicting potential outcomes. This reduces the time and cost involved in developing new medications.
7. Health Monitoring
Wearable devices equipped with NLP analyze speech and text data to monitor health conditions like mental well-being, stress, or even early signs of cognitive decline.
Challenges of NLP in Healthcare
1. Data Privacy
Handling sensitive patient information requires strict compliance with privacy regulations. Ensuring data security is a significant challenge.
2. Complex Medical Language
Medical terminology is diverse and includes abbreviations, synonyms, and jargon. NLP systems must be trained to understand this complexity accurately.
3. Integration with Existing Systems
Integrating NLP tools with legacy healthcare systems can be technically challenging and costly.
4. Bias in Data
NLP models are only as good as the data they are trained on. If the data is biased, the outputs can be misleading.
5. Lack of Standardization
Healthcare data formats vary widely, making it difficult for NLP systems to process information uniformly.
How NLP Addresses Key Healthcare Issues?
1. Reducing Physician Burnout
NLP automates routine documentation tasks, allowing doctors to focus more on patient care.
2. Predicting Disease Outbreaks
By analyzing news articles, social media, and health reports, NLP helps predict and respond to disease outbreaks.
3. Enhancing Mental Health Support
NLP tools analyze speech patterns and text data to detect signs of depression, anxiety, or other mental health issues.
4. Streamlining Administrative Work
NLP automates appointment scheduling, insurance claims processing, and other administrative tasks, increasing overall efficiency.
The Future of NLP in Healthcare
1. Personalized Medicine
NLP will play a significant role in tailoring treatments based on individual patient data, leading to better outcomes.
2. Voice-Activated Assistants
Advanced voice recognition systems will assist doctors in real-time during surgeries or consultations.
3. Advanced Diagnostics
As NLP technologies evolve, they will provide deeper insights into patient data, enabling more precise diagnostics.
4. Global Health Monitoring
NLP will be used to analyze global health data, providing insights into emerging health trends and guiding policy decisions.
Balancing Technical and Human Aspects
While NLP significantly enhances healthcare efficiency, it is essential to maintain a human touch in patient interactions. Technology should complement, not replace, human expertise and empathy in healthcare settings.
Conclusion: What is the importance of NLP in healthcare?
NLP tools have the potential to significantly improve care delivery and streamline operations, but the healthcare sector still has to increase its data capacities before implementing them. Therefore, the key to overseeing clinical decision support and patient health explanations will be NLP and other machine learning methods.
NLP implementation in healthcare initiatives is not a complete answer to every issue. Therefore, the system in this sector must be able to understand the sublanguage that patients and medical professionals utilize.
FAQs: What is the importance of NLP in healthcare?
We have deeply covered each and everything about What is the importance of NLP in healthcare?. However if you have any other queries or questions in your mind about What is the importance of NLP in healthcare? Then dont hesitate to use our contact us page to contact with our team of experts. Our team will be available 24/7.
What is NLP mainly used for in healthcare?
NLP is mostly utilized for text mining in this area of medical science, where algorithms facilitate the rapid discovery of information in patents, clinical trial records, public databases, and other scientific publications.
What is NLP and its importance?
Natural language processing scales other language-related activities and enables computers to converse with people in their native tongue. NLP enables computers, for instance, to read text, hear speech, analyze it, gauge sentiment, and identify key passages.
What is NLP in pharma?
Natural language processing (NLP) is currently impacting the pharmaceutical business as pharma firms are utilizing NLP to help decision making at all phases of drug development and go-to-market, from NLP for data redaction and anonymization of sensitive data, NLP for pharma literature mining,

Everything About Spider Worldwide
You May Also Like
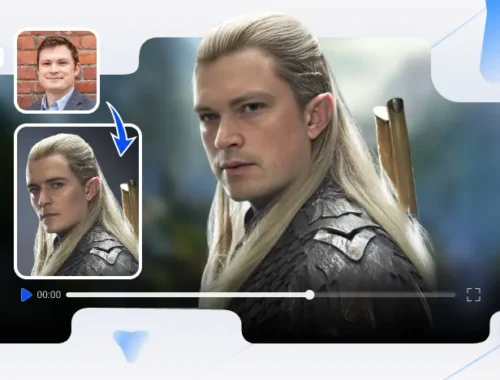
Vidwud AI Dance Generator
2 January 2025
Exploring the Role of Artificial Intelligence in Music Composition and Production
17 January 2025