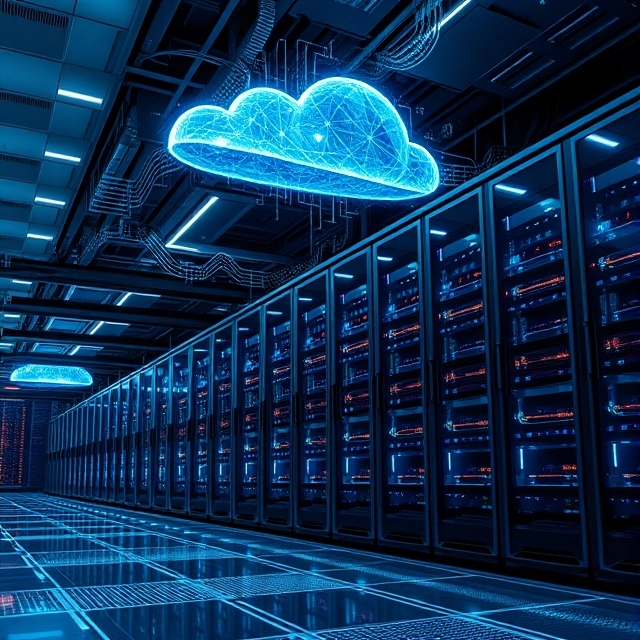
AI Data Storage Solutions for Performance at Scale
Table of Contents
ToggleAI Data Storage Solutions for Performance at Scale: Complete Guide
The exponential growth of artificial intelligence (AI) has ushered in a new era of data complexity and volume. Organizations are now facing the challenge of storing and managing vast amounts of data that AI applications generate and consume. Scalable and efficient data storage solutions have become a paramount concern for businesses looking to leverage AI technologies. Such solutions must not only accommodate the sheer size of data but also ensure its availability and integrity for advanced analytics and machine learning processes. Below, we explore the evolution of data storage in the light of AI demands and how modern solutions are rising to the challenge.
Understanding AI Data Storage Challenges
AI-driven systems generate and process massive datasets, making traditional storage solutions inadequate. The key challenges include:
- High Data Volume: AI workloads require petabytes of data storage to accommodate training datasets and real-time analytics.
- Fast Data Access: AI applications need low-latency solutions to process data efficiently.
- Scalability: As AI models grow, storage solutions must scale seamlessly without compromising performance.
- Data Integrity and Security: Ensuring data reliability and protection against cyber threats is critical.
The Evolution of Data Storage in the AI Era
The evolution of data storage has been significantly influenced by the rise of AI, which demands solutions that can handle diverse data types such as images, audio, and video. Traditional storage systems were designed for transactional purposes, but AI models now require real-time access to large datasets for training and inference. Innovations like all-flash arrays and software-defined storage have been developed to enable quicker data retrieval and processing.
New storage architectures are also embracing distributed and parallel processing frameworks to handle data-intensive workloads typical of AI operations. Data storage for AI is focusing on proximity and latency reduction, enabling AI applications to benefit from reduced delay, enhancing overall system performance.
Characteristics of an Effective AI Data Storage Solution
To be effective, an AI data storage solution must have specific characteristics tailored to AI workflows. These include high-speed performance, scalability, durability, reliability, and interoperability. The storage system should be capable of reading and writing large volumes of data at high speed, and parallel processing to accommodate concurrent operations of AI models.
It should also grow with the increasing amount and complexity of data without experiencing a drop in performance. Built-in mechanisms for data protection, backup, and recovery are essential to ensure data integrity even in the event of hardware failures or cyber incidents. Interoperability is crucial for modern AI data storage solutions, integrating seamlessly with diverse environments and applications, facilitating a harmonious AI ecosystem.
Balancing Speed, Capacity, and Cost in AI Data Storage
The balance between speed, capacity, and cost in AI data storage is a complex task. High-speed storage solutions like SSDs offer AI tasks but come with a higher price, while traditional hard disk drives provide adequate capacity but lack the speed required. Organizations often use tiered storage strategies, using SSDs for performance-critical applications and HDDs for less urgent data storage.
In-memory data storage options also exist, but their cost-effectiveness varies based on the specific AI use case. An organizational approach to data lifecycle management can also help streamline performance and cost efficiencies. Modern compression and deduplication technologies can maximize storage capacity, allowing organizations to store more data economically while maintaining access speeds.
On-Premises vs Cloud Storage Solutions for AI
The debate between on-premises and cloud storage for AI applications centers on control versus scalability. On-premises solutions offer full control, which is crucial for businesses with strict data security and privacy needs. They can also be customized to suit specific AI requirements. In contrast, cloud storage provides flexibility and cost savings with a pay-as-you-go model, and often includes AI data storage solutions like GPU-based instances.
For many organizations, a hybrid approach is the best solution. This combines on-premises and cloud storage, allowing businesses to control sensitive AI data while benefiting from the cloud’s scalability and advanced services. This strategy helps organizations balance data security with the flexibility needed for AI applications.
Ensuring Data Security and Compliance in AI Storage Systems
AI storage solutions must prioritize data security to protect against unauthorized access and breaches. These systems should have robust features like encryption, access controls, and regular security audits. Compliance with regulations like GDPR, HIPAA, and CCPA is crucial for AI applications, and storage solutions must ensure data is properly managed, retained, and can be wiped when no longer needed.
Measures like data anonymization and pseudonymization can enhance privacy while allowing AI systems to learn from valuable datasets. Implementing comprehensive data governance strategies is essential, covering not only storage but also data use and processing within AI applications, aligning with internal policies and external regulatory requirements.
Overall, the optimization of AI data storage solutions is critical in harnessing the full potential of AI technologies. With the correct blend of performance, scalability, cost-efficiency, security, and compliance, organizations can build robust AI infrastructures capable of driving innovation and delivering competitive advantages. As the landscape of AI continues to expand, so will the approaches to storing and managing the lifeblood of its operations.
Types of AI Data Storage Solutions
1. Cloud-Based Storage Solutions
Cloud platforms such as AWS, Google Cloud, and Microsoft Azure provide robust AI storage options. These solutions offer scalability, high availability, and integrated AI tools.
Key Benefits:
- Pay-as-you-go pricing model.
- Global accessibility and redundancy.
- Built-in AI and machine learning tools.
2. On-Premises Storage Solutions
On-premises solutions cater to organizations requiring complete control over their data. These systems often use high-performance storage hardware tailored for AI workloads.
Key Benefits:
- Enhanced security and compliance.
- Tailored performance optimization.
- Reduced dependency on internet connectivity.
3. Hybrid Storage Solutions
Hybrid systems combine the best of cloud and on-premises storage, offering flexibility for organizations with diverse needs.
Key Benefits:
- Customizable based on workloads.
- Balances cost-efficiency and performance.
- Provides a backup solution in case of failures.
Technologies Powering AI Data Storage Solutions
1. NVMe SSDs (Non-Volatile Memory Express Solid-State Drives)
NVMe SSDs deliver high-speed data access, making them ideal for AI applications requiring rapid data processing.
2. Object Storage
Object storage solutions such as Amazon S3 are designed for scalability and are perfect for unstructured data generated by AI workloads.
3. File Storage
File-based storage solutions, like Network-Attached Storage (NAS), support applications requiring high throughput and parallel data access.
4. HDDs with AI Enhancements
Hard disk drives (HDDs) are evolving with AI-specific enhancements to handle cost-effective, high-capacity data storage needs.
Benefits of AI Data Storage Solutions
- Enhanced Scalability: Enables businesses to handle growing datasets without sacrificing performance.
- Improved Data Accessibility: Ensures fast and seamless data access for AI applications.
- Cost Optimization: Solutions like data tiering reduce storage costs by optimizing resource allocation.
- Streamlined AI Workflows: Reduces bottlenecks in data processing pipelines, improving overall efficiency.
Use Cases of AI Data Storage Solutions
1. Healthcare and Medical Research
AI-driven research relies on storage solutions to process and analyze large datasets like medical imaging and genomics data.
2. Autonomous Vehicles
Autonomous vehicles generate terabytes of data daily. Scalable storage solutions ensure real-time data processing for safe navigation.
3. Financial Services
Financial institutions use AI storage solutions for fraud detection, predictive analytics, and customer insights.
4. Media and Entertainment
AI in media relies on high-speed storage for video processing, editing, and distribution.
Future of AI Data Storage Solutions
1. AI-Powered Storage Management
AI will increasingly automate storage management tasks, enhancing efficiency and reducing operational overhead.
2. Edge Computing Integration
Edge storage solutions will enable real-time data processing closer to data sources, reducing latency.
3. Quantum Storage Advancements
Quantum computing developments may revolutionize data storage, offering unprecedented speed and capacity for AI applications.
Conclusion: AI Data Storage Solutions for Performance at Scale
AI data storage solutions are critical for organizations aiming to harness the full potential of AI while maintaining performance at scale. By adopting the right mix of cloud, on-premises, or hybrid storage options and leveraging cutting-edge technologies, businesses can meet their growing data needs efficiently and cost-effectively. Investing in scalable, secure, and high-performance storage solutions ensures a solid foundation for future AI advancements.
FAQs: AI Data Storage Solutions for Performance at Scale
Below are some of the FAQs about AI Data Storage Solutions for Performance at Scale.
How does artificial intelligence store data?
How is data stored by AI? Data is not stored by AI itself. Rather, specialized data storage systems that are built to store and retrieve massive amounts of data quickly are needed for AI applications.
What is AI powered storage?
To increase efficiency and save costs, these storage systems usually use technologies like tiering and data reduction, which includes deduplication and compression. Additionally, AI storage places a high priority on data security, using encryption and access control to protect sensitive information.
How does AI impact data storage needs?
AI applications require vast amounts of data for training and inference, leading to the need for scalable, fast, and reliable storage solutions. Traditional storage solutions are often inadequate, pushing innovations like all-flash arrays and distributed processing architectures.
What is the difference between on-premises and cloud AI data storage?
On-premises solutions offer full control, enhanced security, and tailored performance optimization but require higher upfront costs. Cloud storage, on the other hand, provides scalability, flexibility, and cost savings with a pay-as-you-go model, making it ideal for many AI workloads.
What are the security and compliance requirements for AI data storage?
AI storage solutions must include encryption, access controls, and regular security audits to ensure data protection. Compliance with regulations like GDPR, HIPAA, and CCPA is essential for AI applications, requiring careful data management and privacy practices.
How can organizations ensure data integrity in AI storage systems?
Organizations can ensure data integrity by implementing backup and recovery systems, using high-performance storage with built-in redundancy, and employing data governance strategies to maintain accuracy, reliability, and compliance.
You May Also Like
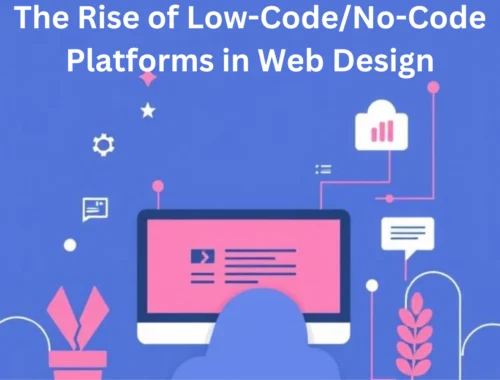
The Rise of Low-Code/No-Code Platforms in Web Design
18 February 2025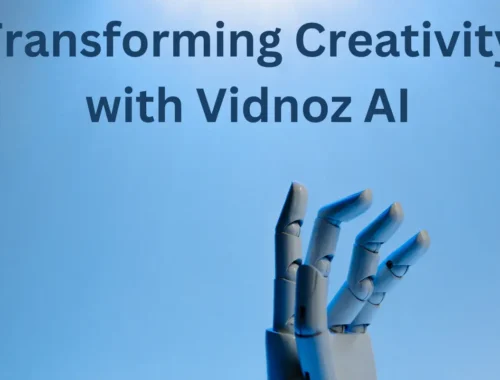
Transforming Creativity with Vidnoz AI
5 January 2025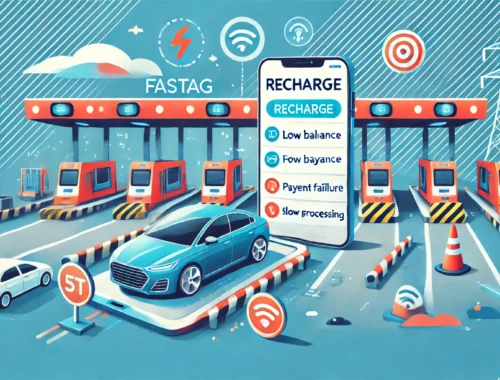